Domain-specific recommendation based on deep understanding of text
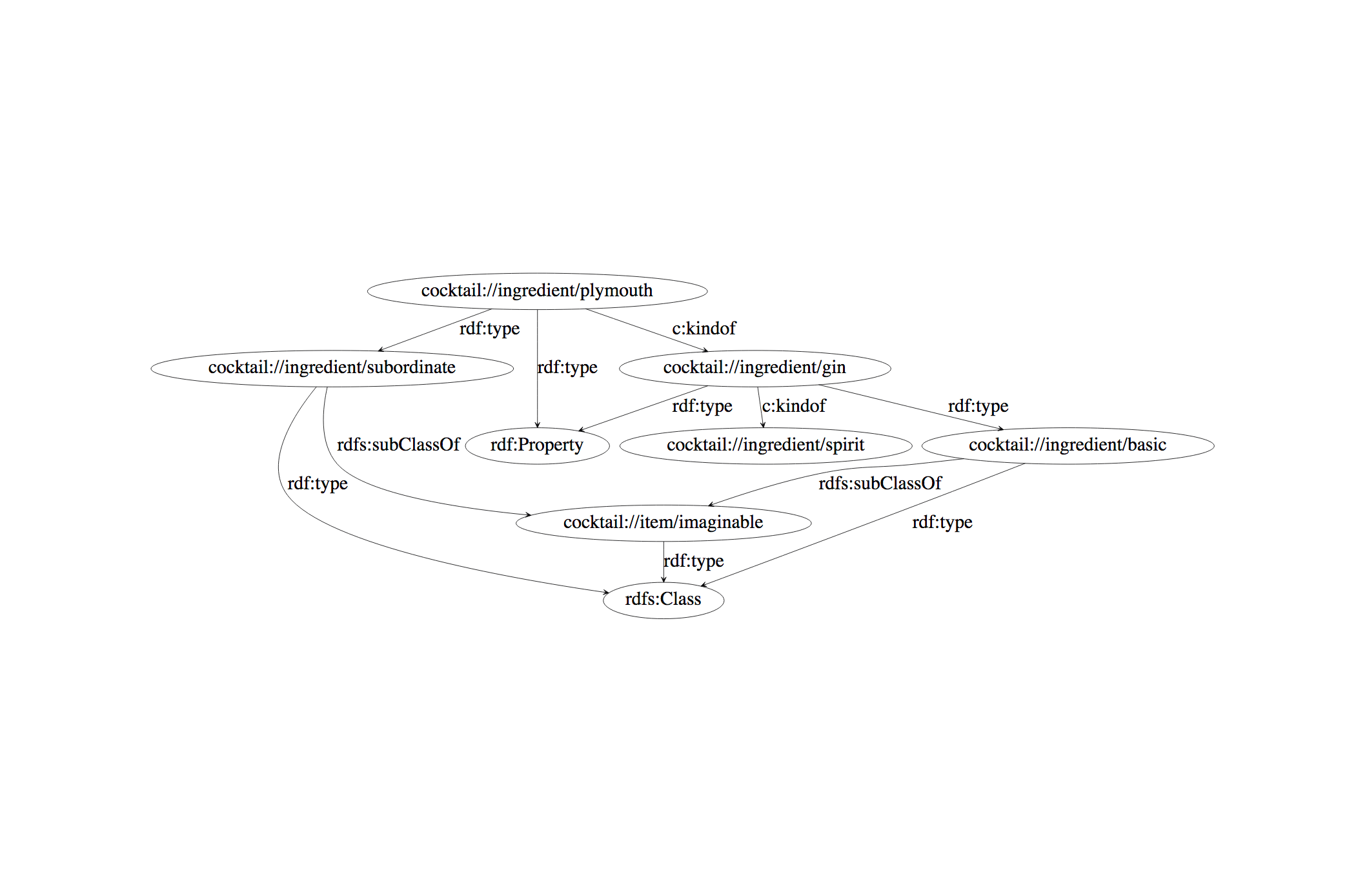
This thesis considers the process of development for a domain-spec recommender system that uses the domain of cocktail recipes for experiments. Based on ontology a deep understanding of text is created — recipes are considered. The ontology is designed by basic categories to extract features such as ingredients. Ingredients are modeled by flavors for comparability. The process of data processing along with the recommendation uses the KDD process as its guidelines. The key of the recommendation is based on domain-specific distance functions. A nearest-neighbor approach is used to classify recommendations for a given favorite. Validation is considered based on the acceptability of domain experts.